Wan VACE: All-in-One Video Creation and Editing
Wan VACE(Wan 2.1 VACE) is an all-in-one model designed for Wan video creation and editing. It encompasses various tasks, including reference-to-video generation (R2V), video-to-video editing (V2V), and masked video-to-video editing (MV2V), allowing users to compose these tasks freely. This functionality enables users to explore diverse possibilities and streamlines their workflows effectively, offering a range of capabilities, such as Move-Anything, Swap-Anything, Reference-Anything, Expand-Anything, Animate-Anything, and more.
Wan2.1 VACE: Three Core Capabilities Analysis
Multi-modal Information Input,Making video generation more controllable.
Traditional video generation workflows, once completed, make it difficult to adjust character postures, actions, scene transitions, and other details. Wan2.1 VACE provides powerful controllable capabilities, supporting generation based on human poses, motion flow, structural preservation, spatial movement, camera angles, and other controls, while also supporting video generation based on themes and background references.
Subject + Background Reference → Video Generation
The core technology behind this is Wan VACE's multi-modal input mechanism. Unlike traditional models that rely solely on text prompts, Wan VACE(Wan2.1 VACE) has built a unified input system that integrates text, images, videos, masks, and control signals.
For image input, Wan VACE(Wan 2.1 VACE) supports object reference images or video frames. For video input, users can use Wan VACE to regenerate content through operations such as erasing and local expansion. For local regions, users can specify editing areas through binary 0/1 signals. For control signals, Wan VACE(Wan2.1 VACE) supports depth maps, optical flow, layouts, grayscale, line drawings, and pose estimation.
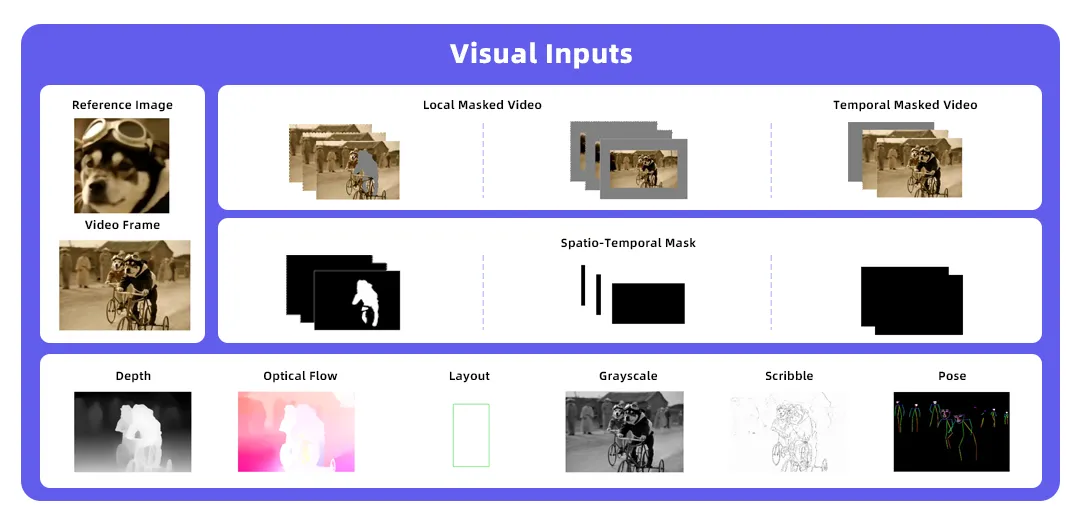
Unified Single Model - One-Stop Solution for Multiple Tasks
Wan VACE(Wan2.1 VACE) supports content replacement, addition, or deletion operations in specified areas within videos. In terms of time dimension, Wan VACE can arbitrarily extend the video length at the beginning or end. In terms of spatial dimension, it supports progressive generation of backgrounds or specific regions, such as background replacement - under the premise of preserving the main subject, the background environment can be changed according to prompts.
Thanks to the powerful multi-modal input module and Wan2.1's generation capabilities, Wan VACE can easily master the functions that traditional expert models can achieve, including:
Image Reference Capability
Given reference subjects and backgrounds, it can achieve consistent element generation
Video Redrawing Capability
Including pose transfer, motion control, structure control, and recoloring
Local Editing Capability
Including subject reshaping, subject removal, background extension, and duration extension
Free Combination of Multiple Tasks - Unleashing AI Creative Boundaries
Wan VACE(Wan2.1 VACE) also supports the free combination of various single-task capabilities, breaking through the limitations of traditional expert models that work in isolation. As a unified model, it can naturally integrate capabilities such as video generation, pose control, background replacement, and local region editing. There's no need to train new models for single-function tasks separately.
This flexible combination mechanism not only greatly simplifies the creative process but also vastly expands the creative boundaries of AI video generation. For example:
Image Reference + Subject Reshaping → Object Replacement in Videos
Motion Control + First Frame Reference → Pose Control of Static Images
Image Reference + First Frame Reference + Background Extension + Duration Extension → Transform Vertical Images into Horizontal Videos with Referenced Elements
Wan VACE: Model Design Highlights
VCU - A More Flexible and Unified Input Format
Through analysis and summarization of input patterns for four common tasks (text-to-video, image-to-video, video-to-video, and partial video-to-video), we have proposed a flexible and unified input paradigm: Video Condition Unit (VCU).
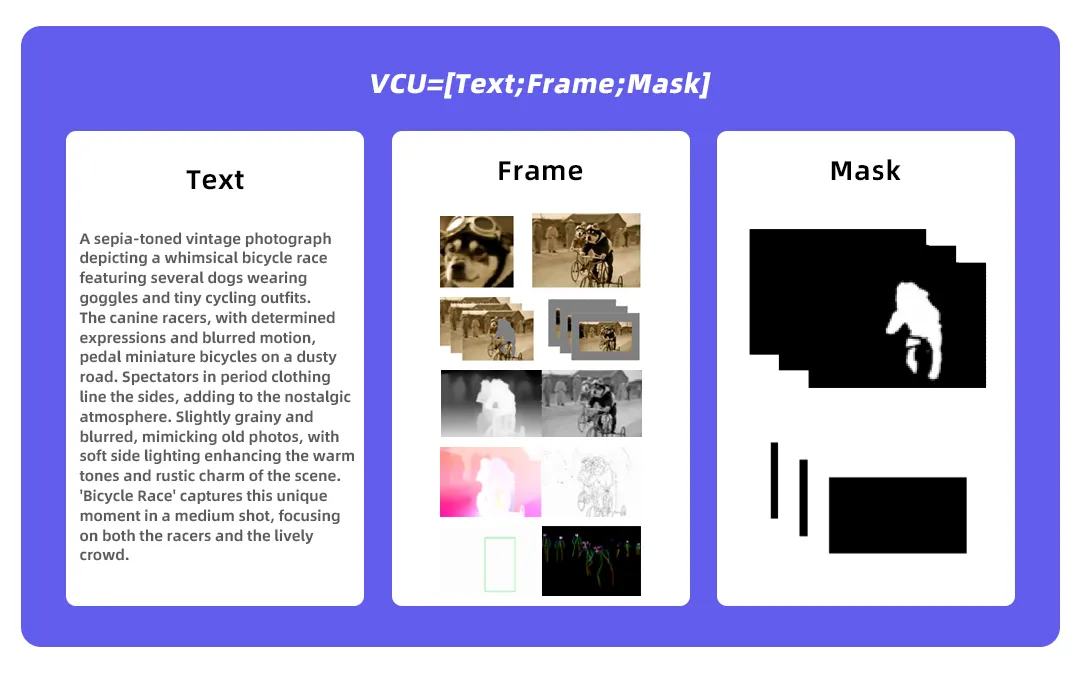
It summarizes various multimodal context inputs into three main forms: text, frame sequences, and mask sequences, unifying the input format for 4 types of video generation and editing tasks. The frame sequences and mask sequences in VCU can be mathematically superimposed, creating conditions for free combination of multiple tasks.
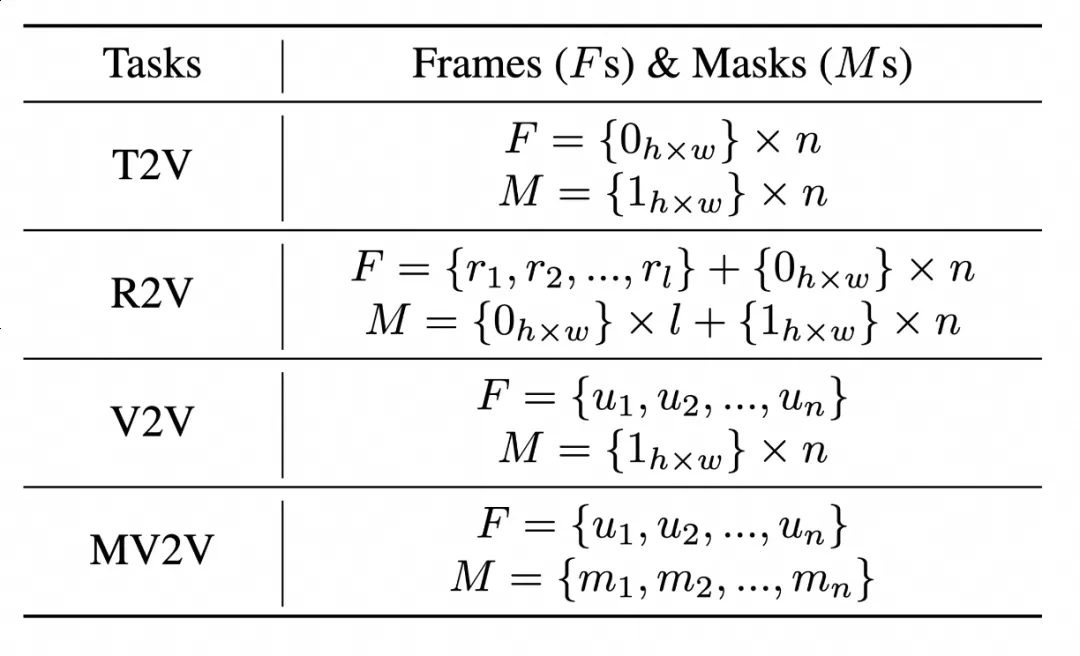
Multimodal Token Serialization - Key to Unified Modeling
How to uniformly encode multimodal inputs into token sequences that can be processed by diffusion Transformers? This is a major challenge that Wan VACE needs to solve.
First, Wan VACE 2.1 conceptually decouples the Frame sequences in VCU input into two categories: one is the RGB pixels that need to be preserved unchanged (invariant frame sequences), and the other is content that needs to be regenerated based on prompts (variable frame sequences). Next, these three types of inputs (variable frames, invariant frames, and masks) are encoded into latent spaces separately. The variable and invariant frames are encoded through VAE into a space consistent with the DiT model's noise dimensions, with 16 channels; while the mask sequences are mapped to latent features with consistent spatiotemporal dimensions and 64 channels through transformation and sampling operations.
Finally, the latent space features of Frame sequences and mask sequences are combined and mapped to DiT token sequences through trainable parameters.

Context Adapter Fine-Tuning - Efficient Training Strategy
In terms of training strategy, we compared two approaches: global fine-tuning and context adapter fine-tuning. Global fine-tuning, which involves training all DiT parameters, can achieve faster inference speed. The context adapter fine-tuning approach, on the other hand, keeps the original base model parameters fixed and selectively copies and trains some of the original Transformer layers as additional adapters.
Experiments show that while both approaches have similar validation losses, context adapter fine-tuning offers faster convergence speed and avoids the risk of losing basic capabilities. Therefore, this open-source version adopts this method for training.
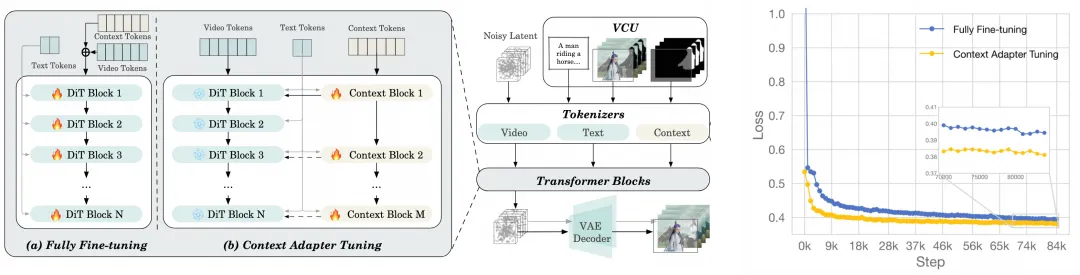
Through the quantitative evaluation of the Wan VACE 2.1(Wan2.1 VACE) series models released this time, it can be seen that compared to the 1.3B preview version, the model has a significant improvement in multiple key indicators.
Community Works
If your work has improved Wan2.1 and you would like more people to see it, please inform us.
Phantom has developed a unified video generation framework for single and multi-subject references based on Wan2.1-T2V-1.3B. Please refer to their examples.
UniAnimate-DiT, based on Wan2.1-14B-I2V, has trained a Human image animation model and has open-sourced the inference and training code. Feel free to enjoy it!
CFG-Zero enhances Wan2.1 (covering both T2V and I2V models) from the perspective of CFG.
TeaCache now supports Wan2.1 acceleration, capable of increasing speed by approximately 2x. Feel free to give it a try!
DiffSynth-Studio provides more support for Wan2.1, including video-to-video, FP8 quantization, VRAM optimization, LoRA training, and more. Please refer to their examples.
VACE Wan 2.1 is an upcoming new model from Wan AI. The open-source release conference has already been held, and the model will be officially released soon. Stay tuned for more updates and exciting features!